Posted At: Mar 03, 2025 - 635 Views
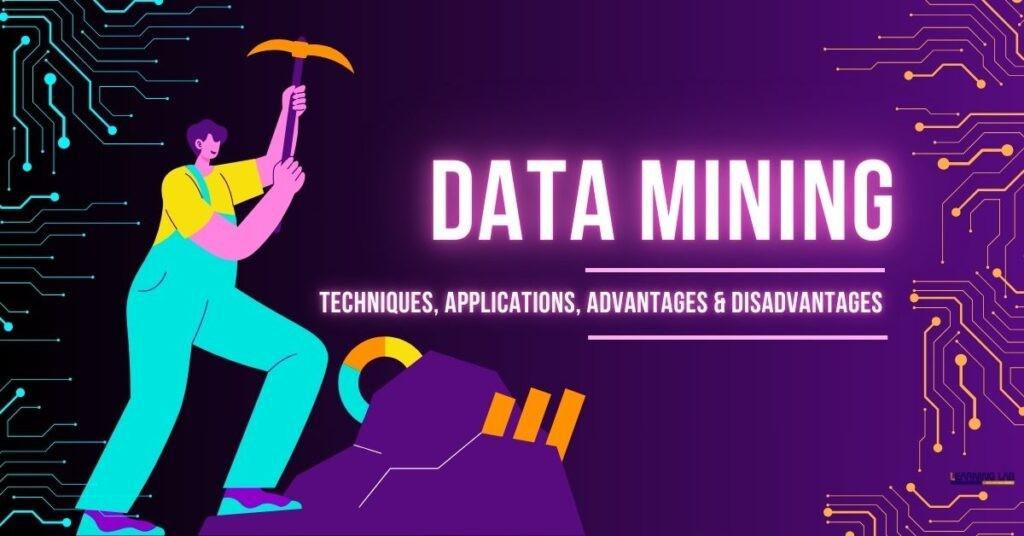
Introduction
As transportation networks evolve, so do the methods of data collection and analysis. Traditional manual data collection methods, such as fare counting and paper-based ticketing, are no longer sufficient for modern transit optimization. The shift toward automated data mining technologies has enabled real-time decision-making, improved efficiency, and enhanced passenger experiences.
This study explores the challenges of manual data collection, the limitations of conventional transportation data systems, and the benefits of transitioning to automated data mining technologies.
π Download the Full Report (PDF): Click Here
1. Manual Data Collection in Transportation
π Key Insight: Manual data collection methods were once the foundation of transit operations but lacked scalability and real-time insights.
πΉ Traditional Data Collection Methods:
β Fare Counting & Ticket Recording: Conductors manually recorded revenue and passenger counts.
β Periodic Manual Surveys: Passenger demographics and travel patterns were collected through onboard interviews.
β Paper-Based Ticketing: Ticket sales and usage were logged manually, creating a record of transit activity.
πΉ Limitations of Manual Methods:
β High potential for human error in fare counting and ticket validation.
β Limited data coverageβonly periodic snapshots rather than continuous tracking.
β Slow data processing, leading to outdated insights and delayed decision-making.
π‘ Takeaway: Manual data collection lacked accuracy, timeliness, and the ability to handle complex transit networks, leading to inefficiencies in route planning and passenger management.
2. Why Manual Data Collection is No Longer Sufficient
π Key Insight: The complexity of modern transportation networks demands real-time, automated data collection for operational efficiency.
πΉ Challenges with Manual Data Collection:
β High Error Rates: Human data entry errors result in inaccurate transit statistics.
β Limited Decision-Making Power: Slow data processing hinders real-time adjustments to schedules and routes.
β Scalability Issues: Growing urban populations require more dynamic transit planning.
πΉ Why Automated Data Collection is the Future:
β Enables instant data access for more informed decision-making.
β Reduces human labor costs and minimizes errors.
β Supports data-driven route optimization, leading to improved passenger experiences.
π‘ Strategic Takeaway: Cities must transition to automated data mining techniques to ensure scalable, accurate, and real-time transit management.
3. Case Study: The Impact of Data Mining on Transit Optimization
π Key Insight: Upgrading from manual to automated data collection enhances operational efficiency, service reliability, and cost-effectiveness.
πΉ Findings from Capri (2015) & Vernizzi & Bontempi (2020):
β Optimized Decision-Making: Real-time data enables faster route adjustments.
β Improved Service Reliability: Data-driven insights reduce transit delays and inefficiencies.
β Cost & Time Savings: Automation reduces the need for labor-intensive ticketing and surveys.
πΉ Challenges in Transitioning to Automated Systems:
β Requires significant initial investment in infrastructure and technology.
β Staff training is necessary for adapting to new digital data collection methods.
β Maintenance and system upgrades demand ongoing financial support.
π‘ Policy Insight: Despite the costs, transitioning to automated transit data collection enhances long-term efficiency and service quality.
4. The Future of Data Mining in Transportation Systems
β AI & Machine Learning Integration: Predictive analytics will optimize route planning and passenger load distribution.
β IoT & Real-Time Sensors: Smart transportation systems will provide real-time insights into road conditions and vehicle performance.
β Automated Fare Collection & Mobile Payments: Digital transactions will replace traditional paper-based ticketing.
π‘ Best Practice: Transit agencies must invest in smart data collection technologies to improve urban mobility and passenger satisfaction.
Conclusion
The shift from manual to automated data collection is essential for modernizing transportation systems, reducing inefficiencies, and optimizing transit networks. By integrating data mining technologies, real-time analytics, and AI-driven automation, cities can enhance decision-making, improve passenger experiences, and future-proof public transit infrastructure.
π₯ Download Full Report (PDF): Click Here
Related Data Science & Transportation Analytics Resources π
πΉ How Big Data is Revolutionizing Public Transportation
πΉ Automated Fare Collection: The Future of Transit Payments
πΉ Real-Time Data Mining for Smarter Cities
π Need expert insights on data mining and transportation analytics? π Our professional writers at Highlander Writers can assist with AI-driven transit research, data analytics projects, and transportation optimization studies!
π― Hire a Writer Today!
Leave a comment
Your email address will not be published. Required fields are marked *