Posted At: Mar 03, 2025 - 635 Views
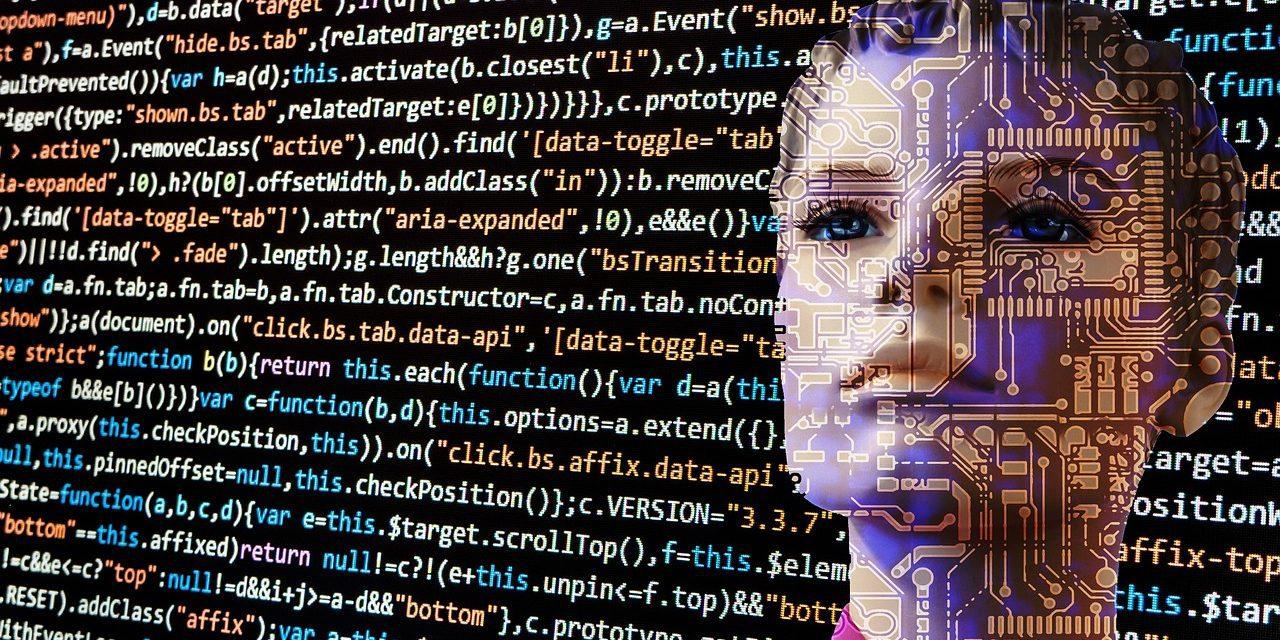
Introduction
Social media platforms have transformed how people connect, share information, and engage with digital content. However, they also collect vast amounts of user data, which is analyzed using computational intelligence (CI) techniques such as machine learning, sentiment analysis, and predictive modeling.
This study explores how social networking platforms gather data, the ethical concerns of data usage, and the role of computational intelligence in extracting valuable insights.
🚀 Download the Full Report (PDF): Click Here
1. How Social Media Platforms Collect User Data
📌 Key Insight: Social media platforms use advanced algorithms to track user interactions, preferences, and engagement patterns.
🔹 What Data is Collected?
✔ User Connections & Demographics – Friends, followers, age, location, and interests.
✔ Engagement Metrics – Likes, shares, comments, and interaction history.
✔ Behavioral Data – Browsing habits, ad clicks, and content preferences.
✔ Geographic Information – Real-time location tracking through mobile apps.
🔹 Example: Facebook’s Data Collection
✔ Facebook monitors user interactions to build detailed profiles.
✔ AI algorithms analyze engagement patterns to deliver personalized recommendations.
💡 Takeaway: Social networking platforms gather extensive data to refine user experience and improve targeted advertising.
2. Ethical Concerns: The Benefits and Risks of Social Media Data Usage
📌 Key Insight: While social media data enhances user experience, it also raises privacy concerns.
🔹 Potential Benefits of Social Media Data:
✔ Personalized Content & Recommendations – Users receive customized articles, events, and groups.
✔ Community Building – Algorithms connect people with similar interests.
✔ Improved Advertising Efficiency – Businesses reach highly relevant audiences.
🔹 Potential Risks of Social Media Data Usage:
✔ Targeted Manipulation & Misinformation – Social media can reinforce biases and limit diverse perspectives.
✔ Filter Bubbles & Echo Chambers – Users only see content that aligns with their existing beliefs.
✔ Privacy Violations – Unauthorized data sharing raises security concerns.
💡 Strategic Insight: Social media companies must balance innovation with ethical responsibility to protect user privacy.
3. The Role of Computational Intelligence in Social Networking
📌 Key Insight: Machine learning and data mining techniques help analyze social media data for insights.
🔹 Computational Intelligence Techniques Used in Social Media Analytics:
✔ Sentiment Analysis – AI scans posts and comments to determine public opinion.
✔ Trend Detection – Identifies emerging topics based on viral discussions.
✔ User Behavior Prediction – Forecasts engagement levels and interaction patterns.
✔ Anomaly Detection – Detects suspicious activities such as fake accounts or misinformation campaigns.
🔹 Example: How CI Enhances Social Media Algorithms
✔ Sentiment analysis determines how users feel about brands, products, or policies.
✔ Predictive models estimate which posts will gain the most engagement.
✔ Fraud detection systems flag suspicious user activities for security monitoring.
💡 Takeaway: Computational intelligence transforms raw social media data into actionable insights for businesses, researchers, and security analysts.
4. Case Studies: How AI is Revolutionizing Social Media Analytics
📌 Case Study 1: Sentiment Analysis for Brand Monitoring
✔ Companies analyze social media sentiment to understand customer satisfaction.
✔ AI detects positive vs. negative feedback to refine marketing strategies.
📌 Case Study 2: Fake News Detection Using CI
✔ AI models scan social media for misinformation and bot activity.
✔ Machine learning filters false claims and prevents the spread of fake news.
📌 Case Study 3: Predicting Virality of Social Media Posts
✔ Neural networks predict which posts are likely to go viral based on engagement history.
✔ Helps brands optimize content strategy for better audience reach.
💡 Industry Insight: Businesses and researchers can leverage AI-powered social media analytics to drive marketing success, security improvements, and audience engagement.
5. Practical Applications of CI in Social Networking
✔ Social Media Marketing: AI-driven analytics optimize content strategies and advertising campaigns.
✔ Cybersecurity & Fraud Prevention: Machine learning detects fake accounts and malicious activity.
✔ Political & Social Research: Sentiment analysis gauges public opinion on key issues.
✔ Customer Experience Enhancement: Predictive models improve user recommendations and engagement.
💡 Best Practice: Businesses should integrate CI-powered analytics into their marketing and security strategies for a competitive advantage.
Conclusion
The fusion of social networking and computational intelligence enables advanced data-driven insights for businesses, researchers, and policymakers. By applying machine learning, sentiment analysis, and predictive modeling, companies can enhance user engagement, detect security threats, and refine digital marketing strategies.
📥 Download Full Report (PDF): Click Here
Related AI & Social Media Analytics Resources 📚
🔹 How AI is Transforming Social Media Marketing
🔹 The Role of Sentiment Analysis in Brand Reputation Management
🔹 Cybersecurity & Fake News Detection in Social Media
📌 Need expert insights on AI-powered social media analytics? 🚀 Our professional writers at Highlander Writers can assist with social media research, machine learning applications, and data science projects!
🎯 Hire a Writer Today!
Â
Leave a comment
Your email address will not be published. Required fields are marked *