Posted At: Mar 03, 2025 - 633 Views
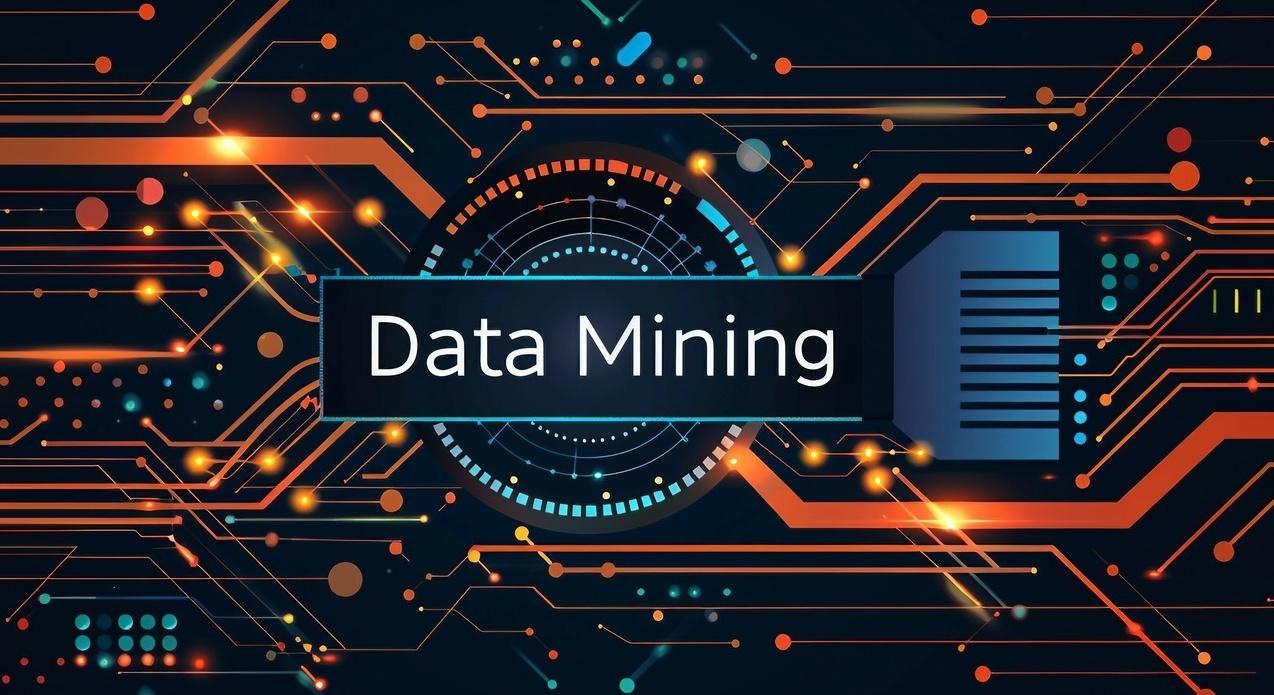
Introduction
Data classification is a fundamental technique in data mining and machine learning, enabling computers to recognize patterns, categorize information, and make predictions. Applications range from image recognition and fraud detection to spam filtering and medical diagnosis.
This study explores classification techniques, decision tree algorithms, hyperparameter tuning, and best practices for model evaluation to enhance data mining efficiency.
π Download the Full Report (PDF): Click Here
1. What is Data Classification?
π Key Insight: Data classification divides datasets into meaningful categories, improving decision-making and predictive analytics.
πΉ Applications of Data Classification:
β Image Recognition: Identifies objects in photos and videos.
β Spam Filtering: Differentiates between spam and legitimate emails.
β Medical Diagnosis: Predicts disease risks based on patient data.
πΉ How Classification Works:
β The algorithm learns from labeled datasets to recognize patterns.
β Once trained, the model classifies new, unseen data into predefined categories.
π‘ Takeaway: Data classification improves accuracy in automated decision-making systems across various industries.
2. General Framework for Classification Models
π Key Insight: Effective classification models follow a structured framework for data preparation, training, and testing.
πΉ Steps in the Classification Process:
β Data Preprocessing: Cleaning, transforming, and handling missing values.
β Model Training: Using labeled datasets to teach the algorithm.
β Testing & Validation: Evaluating the modelβs generalization ability on new data.
πΉ Example: Image-Based Classification Models
β A machine learning model trained on thousands of images can classify cats vs. dogs in photos.
β The model learns pixel patterns and improves through feedback loops.
π‘ Best Practice: A well-trained model minimizes errors and enhances prediction accuracy in real-world applications.
3. Decision Trees & Model Modifications
π Key Insight: Decision trees are widely used for their interpretability, flexibility, and efficiency in classification tasks.
πΉ What is a Decision Tree?
β A flowchart-like model that splits data based on decision rules.
β Nodes represent features, branches represent decision paths, and leaves represent final classifications.
πΉ Enhancing Decision Tree Performance:
β Pruning: Removes unnecessary branches to prevent overfitting.
β Boosting: Combines multiple weak models to create a stronger classifier.
π‘ Takeaway: Decision trees are highly interpretable, making them ideal for finance, healthcare, and fraud detection applications.
4. Understanding Hyperparameters in Model Optimization
π Key Insight: Hyperparameters affect model performance and must be carefully tuned for optimal results.
πΉ Examples of Key Hyperparameters:
β Learning Rate: Controls how quickly a model updates weights.
β Tree Depth (for Decision Trees): Determines model complexity and generalizability.
β Number of Estimators (for Boosting Models): Affects prediction strength.
πΉ Hyperparameter Tuning Techniques:
β Grid Search: Tests multiple parameter combinations to find the best fit.
β Random Search: Selects hyperparameters randomly to improve efficiency.
β Bayesian Optimization: Uses probabilistic models to fine-tune performance.
π‘ Best Practice: Adjusting hyperparameters correctly prevents underfitting and overfitting, leading to better accuracy and efficiency.
5. Common Pitfalls in Model Selection & Evaluation
π Key Insight: Overfitting and poor evaluation metrics can lead to misleading results in machine learning models.
πΉ Key Pitfalls in Model Training:
β Overfitting: When a model performs well on training data but fails on real-world data.
β Poor Validation Techniques: Using biased datasets that donβt reflect real-world conditions.
β Misleading Accuracy Scores: High accuracy doesnβt always mean better performance, especially with imbalanced datasets.
πΉ How to Improve Model Evaluation:
β Cross-Validation: Splits data into training and validation sets for unbiased testing.
β Balanced Metrics: Use F1-score, precision, recall, and AUC-ROC curves instead of just accuracy.
β Continuous Model Updates: Regularly update models with new data to maintain effectiveness.
π‘ Takeaway: Proper model evaluation ensures reliability and reduces the risk of inaccurate predictions.
6. Practical Applications of Data Classification
β Finance: Fraud detection models classify transactions as legitimate or fraudulent.
β Healthcare: AI systems predict disease diagnoses based on patient data.
β E-commerce: Recommender systems classify products based on customer preferences.
π‘ Best Practice: Combining decision trees, hyperparameter tuning, and proper evaluation metrics leads to more accurate and efficient classification models.
Conclusion
Data classification is a core technique in data mining, allowing businesses and researchers to categorize information, make predictions, and enhance decision-making. Using decision trees, hyperparameter tuning, and robust evaluation strategies, machine learning models can improve accuracy, efficiency, and real-world applicability.
π₯ Download Full Report (PDF): Click Here
Related Data Science & Machine Learning Resources π
πΉ How Decision Trees Improve Machine Learning Models
πΉ The Role of Hyperparameter Tuning in AI Optimization
πΉ Best Practices for Evaluating Machine Learning Models
π Need expert guidance on data mining and AI? π Our professional writers at Highlander Writers can assist with machine learning projects, data classification research, and algorithm development!
π― Hire a Writer Today!
Β
Leave a comment
Your email address will not be published. Required fields are marked *